2022 Speakers
SPEAKERS

Prof. Kaoru Arakawa
Meiji University, Japan
Kaoru Arakawa received the B.E., M.E. and PhD degrees in Electronic and Electric Engineering from the Univ. of Tokyo in 1980, 1982, and 1986, respectively. During 1984-1985, she studied at Caltech, USA, as a Fulbright Student. In 1989, she joined the Department of Computer Science, Meiji University, as a Full-time Lecturer, and became an Associate Professor, and a Professor in 1992 and 1998, respectively. In 2013, she moved to School of Interdisciplinary Mathematical Sciences, Meiji University, where she is now a Dean and a Professor. In 1995, she was a visiting researcher at the University of California at Santa Barbara. She is a Fellow of IEICE and JFES. She received Best Paper Award from IEICE in 2004, IEEE Tokyo Section Women Pioneer Award in 2006, and SCAT President Grand Award in 2022. Her research interests include audio and visual signal processing, machine learning, and biomedical information analyses.
.
Title : COMBINATION OF GAN WITH INTERACTIVE EVOLUTIONARY COMPUTATION TO DESIGN OBJECTS
Generative adversarial networks (GANs) are a powerful tool for image generation. It can generate realistic images, being helpful to design shapes and colors of objects in images. However, since the object is designed automatically by computation, it is hard to reflect the user’s preference in the design. On the other hand, interactive evolutionary computation (IEC) is well known as a technique which can reflect the user’s preference in designing objects on computers. In IEC, the parameters of the shapes and colors of objects are represented as numerical series, which correspond to chromosomes in genetic algorithm (GA), and are optimized on the basis of the user’s subjective assessment by human interaction. Thus, object design can be realized more powerfully by combining GAN with IEC; GAN can create realistic images of wide-variety of object designs, while IEC can consider the users preference in the design. The combination is performed by optimizing the latent variables in GAN by IEC. A vector of random latent variables is utilized in GAN to generate various images. The generated image depends on the values of the latent variables, thus preferable design image can be obtained by adjusting the values of them. IEC can effectively adjust the values by regarding them as genes in chromosomes, based on the user’s selection.
Recently, some methods were proposed to design objects and artistic images using the combination of GAN and IEC [1]-[5]. In this talk, three of them, which were proposed by the speaker, are introduced. The first one adopts conditional GAN with the input contour images of objects such as shoes [3], the second adopts DCGAN to design tile shapes which reflect the adjectives the user wants to express [4], and the last adopts StyleGAN to design movie posters with letters which consider fine to coarse structure of the contents [5]. Compared with the other works [1][2], our methods have characteristics that some constraints are imposed on the system in order to get satisfactory design result efficiently. Computer simulations show that the proposed systems can generate realistic fine designs, and also the results of subjective assessment verify that the obtained designs are satisfactory enough.
.

Dr. Dong-Sun Kim
Vice President, Korea Electronics Technology Institute, Korea
Dong-Sun Kim received B.E and M.E degrees in the School of Electronics and Electrical Engineering in 1997 and 1999, respectively, from INHA University, Incheon, Korea. In 2005, he received a Ph.D. degree from the School of Information and Telecommunication Engineering of INHA University, Incheon, Korea. Since 1999, he has been with the Korea Electronics Technology Institute (KETI), Gyeonggi-do, Korea and working on R&D at the Semiconductor and Display R&D Division, where he currently is a vice president. In 2018, 2019 and 2020, he joined the Korea Evaluation Institute of Industrial Technology(KEIT) as a semiconductor program director (PD). His research interests are in the areas of wireless/wired communication systems, wireless sensor networks, VLSI & SoC design, multimedia codec design, computer architecture, and embedded system design.
.
Title : A Latency-aware Memory Scheduling Architecture of Neural Network Processor for Deep Learning Systems
This paper presents a comprehensive improved performance of the hardware realizations of artificial neural network (ANN) models, known as hardware neural networks (HNN) with a latency-aware memory scheduler. We study the overall progress in the field across all major ANN models, hardware design approaches. And then, this paper presents a high performance neural network processor optimized for scientific computations such as image processing and artificial neural networks. The proposed processor employs an SIMD architecture consisting of 256 processing units (PUs), a non-linear functional unit (NFU), and a control unit (CU), which are connected through two global data buses, one control bus, and a fully connected matrix programmable bus architecture. The instruction program is stored in the embedded program memory; on the other hand, the data are distributed in the embedded local memories (LMs) and an external data memory. The global data bus and ring bus allow data to broadcast and PU-to-PU data to transfer. The CU generates the control signals for all PUs and allows address jump and branch functions. The NFU is a look-up table memory that realizes an arbitrary non-linear function. Global Register File (GRF) is used to store data from NFU. The data in GRF are to be broadcasted to PUs through the data bus or the ring bus. Each PU consists of 32-bit fixed point numerical arithmetic units, a 32-bit 16-word register file, 16-bit 1.5K-word LM, special purpose registers (CR, FR, and AR), and an address modifier (AM), respectively. The functionality and the performance of the proposed processor are verified with the character recognition application on the FPGA platform board. Finally, we show that neural network processor produces significantly better results on applications accompanied by heavy memory access than software processing. Suppose the proposed processor is implemented as a chip using 0.18-micron process technology, it is expected to operate at 400MHz clock speed, and then its computing power could be over 4 times faster than a 2.8 GHz PC.
.
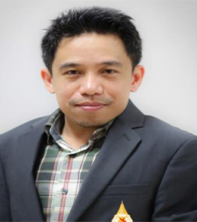
Prof. Nattapol Aunsri
Mae Fah Luang University, Thailand
Nattapol Aunsri received B.Eng. and M.Eng. degrees with Electrical Engineering in Khon Kaen University and Chulalongkorn University in 1999 and 2003, respectively. Also, he received M.Sc. and Ph.D. in Mathematical Sciences in 2008 and 2014, respectively from New Jersey Institute of Technology, USA. Currently he is an assistant Professor of Computer Engineering at the School of Information Technology, Mae Fah Luang University. He was Program Committee Member of IEEE The 21st International Symposium on Wireless Personal Multimedia Communications (WPMC-2018), IEEE The 6th Global Wireless Summit (GWS2018), IEEE ECTI-CON 2018, ISFT 2018, ECTI-DAMT NCON 2021, and ECTI-DAMT NCON 2022. His research areas and expertise are Signal processing, Bayesian filtering, underwater acoustics, biomedical signal processing, machine learning applications, and mathematical and statistical modelling.
.
Title : Model-Based Bayesian Signal Processing
Bayesian signal processing has attracted the reserchers enormously since the last three decades. In order to implement this framework, mathematical models that describe the physical characteristic of the problem are required. The well known filter for this framework is the Kalman filter (KF) that works effectively for the problems under the linear and Gaussian assumptions. However, most real word problems do not follow this restriction, therefore more sophisticataed filters have been proposed. Particle filtering, a sequential Monte Carlo method, is a powerful method for estimating states from non-linear/non-Gaussian models have come into consideration. Particles, which are sets of random samples, are randomly generated to approximate the posterior probability density function (PDF) of the state parameters. Its utilization has been found to be excellent for wide range of aplications. This talk introduces the importance of model-based signal processing for engineering applications, and then discusses how KF and particle filter (PF) can be emplyed for signal processing problems. Some examples from recent reserarches are provided to further elaborate the utilization of the framework